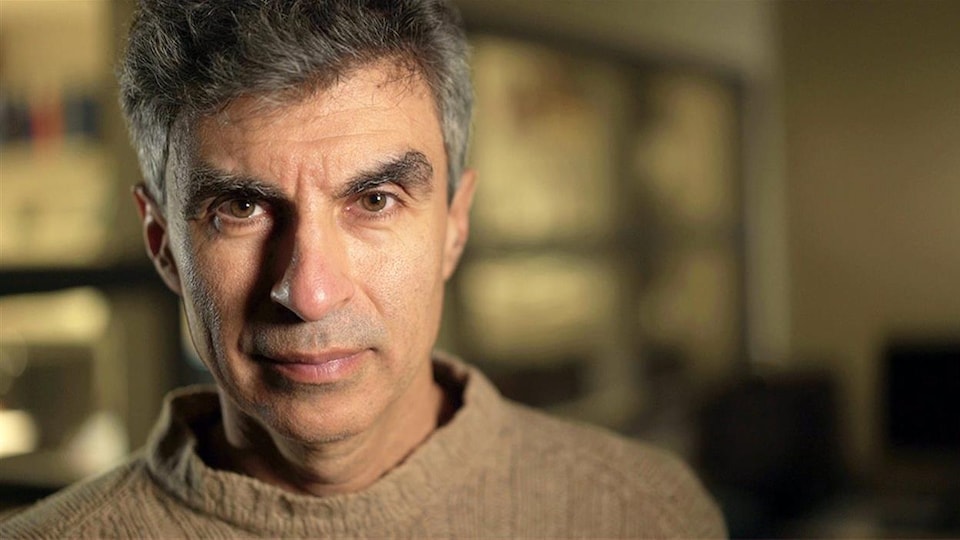
The brain can learn in many different ways, including the hippocampus. The development distributional statistical learning is more dependent upon the hippocampus. It is not clear which brain region plays the most significant role in this process. This article will discuss the differences among the brain regions involved with statistical learning. Here are some examples of the ways our brain learns. In addition to learning by observation, we can learn through experiments.
Behaviorally
The behaviorally-learn statistical learning can help people recognize patterns in themselves and predict the behavioural patterns of others. Adults who are behaviourally able may be better at anticipating the actions and intentions of others. ASD-afflicted adults might have higher statistical learning abilities than other children. These abilities might help them engage more in reciprocal social interactions. However, more research is required to understand how such learning happens.
Although most research in this area has focused on auditory statistical learning, it is becoming increasingly clear that this capability also extends to the visual domain. Infants as young as two months old have been found to learn to identify statistical patterns in visually presented shapes. In one experiment, infants were given a series coloured shapes and taught to identify patterns within the sequences. Children learned more statistically when two-shape set were presented together.
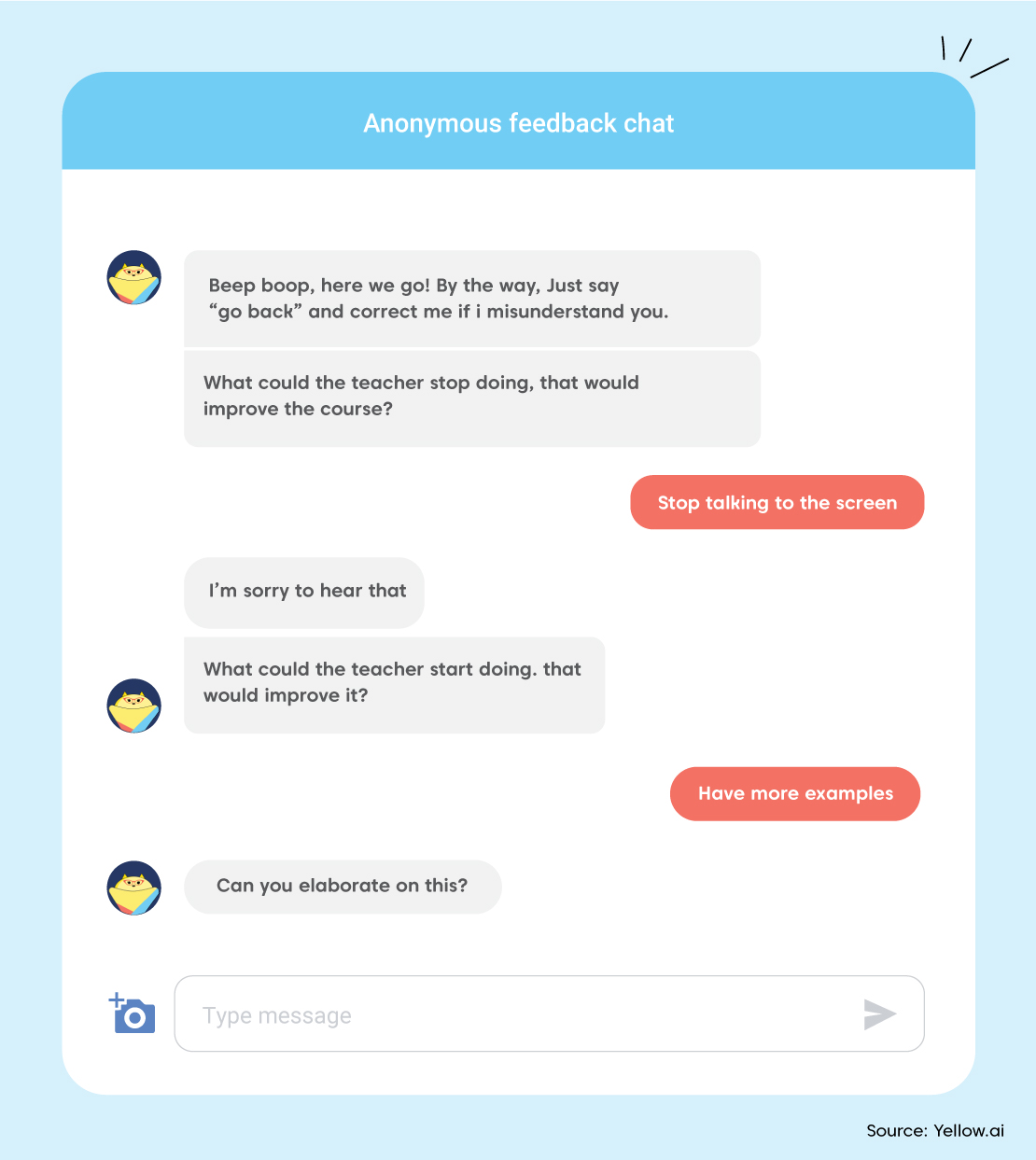
Cognitively
Numerous studies have demonstrated that the brain can cognitively learn statistical patterns and associated associations. This ability is widespread across all ages and gets more sophisticated with age. Adults are particularly adept at acquiring the underlying structure of experiences. They can learn how to process sensory inputs in various modalities and to recognize patterns in physical forces. Statistical learning allows you to extract multiple sets of regularities simultaneously, without interference. It allows us to form spatial and conceptual schemas, and generalized semantic information.
Despite the possibility that statistical learning could be domain-specific it is first discovered in language acquisition. Participants were trained by Johnson Johnson Aslin, Newport and Aslin to recognize statistical probabilities that are associated with musical notes. During training, participants were exposed to a stream of musical tones as a single unit, which they then recognized as a single unit when tested. In a related study, Saffran et al. (1999). Both infants (and adults) learned to recognize the statistical probabilities and musical tones.
Neurologically
There is no one explanation for how statistics help us learn new information. Many theories suggest there may be some neural substrate that controls learning and memory. This theory highlights the role of memory in the creation of memories and how similarity-based activation occurs in both distributional and conditional statistical learning. It also emphasizes the importance and differences between explicit, implicit, and mixed memory.
Regardless of the mechanism involved, there is substantial evidence that there is a combination of domain-general and modality-specific components to SL. Both modality-specific and domain-specific computations produce domain-general principles. Modality-specific information generated during initial encoding is used to further process the data in multimodal regions. Consolidation may allow information from multiple domains to be processed in the brain networks. This allows for similar processing demands.
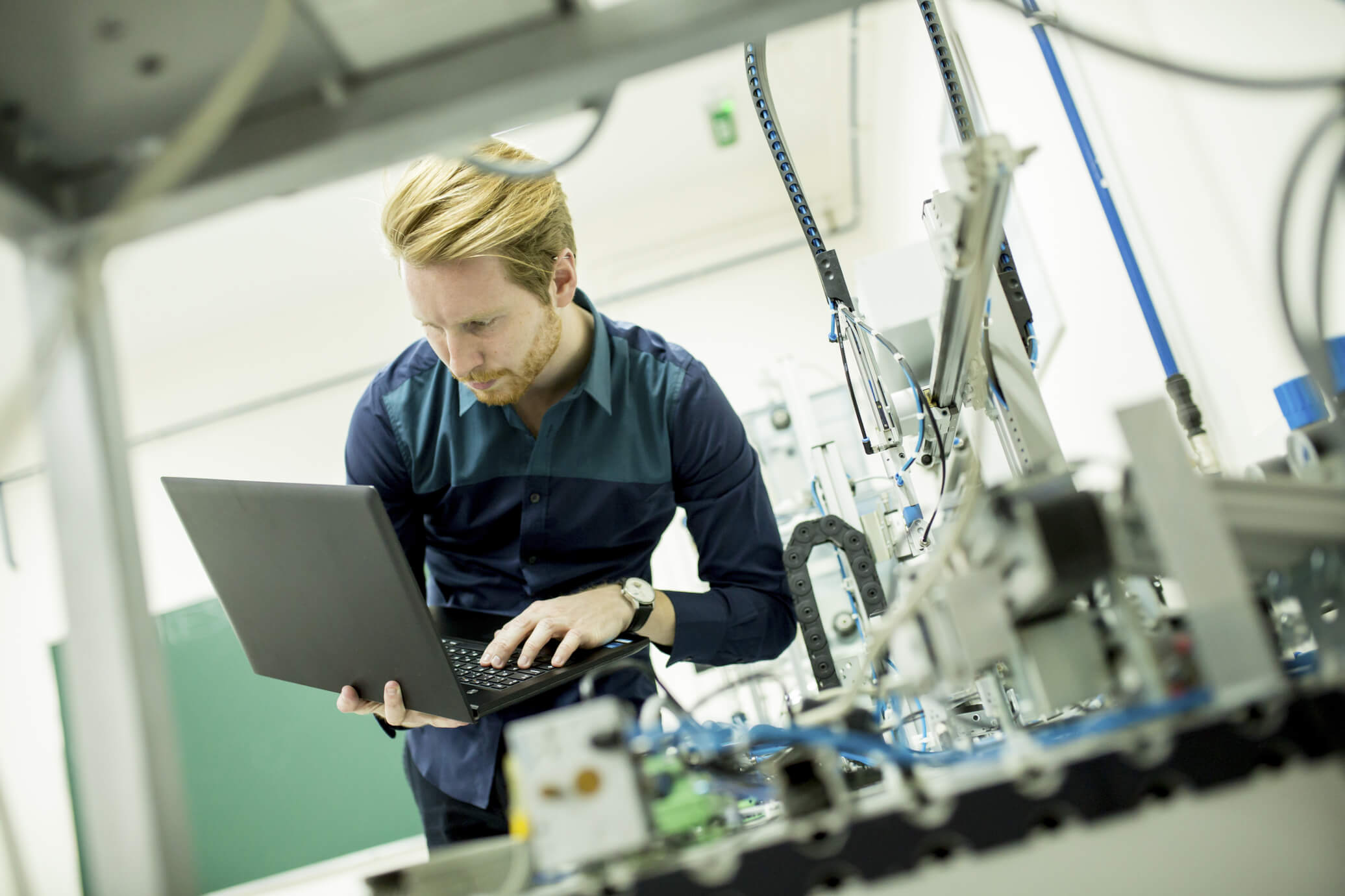
In social interactions
Statistical Learning is the process where people learn from others and draw their own statistics. This process relies on the extraction of input from memory traces and its integration across them. When making decisions, learners are more aware of the frequency and variability in exemplars. This may help them to mitigate the disadvantages that come with low socioeconomic status households. To solve social interaction problems, it is important that people develop a statistically-based reasoning process.
Language development is influenced by statistical learning. Statistics learning is a major factor in the acquisition of language by children. Although socioeconomic status has an effect on language development but it modifies this relationship, A person's level of statistical literacy predicted their performance in grammatical tasks with passive and object relation clauses. It is important to fully understand the role statistical learning plays in language acquisition. However, in order to fully understand how statistical learning influences language development, we must understand the way it works.
FAQ
What is the latest AI invention
Deep Learning is the latest AI invention. Deep learning is an artificial intelligent technique that uses neural networking (a type if machine learning) to perform tasks like speech recognition, image recognition and translation as well as natural language processing. Google developed it in 2012.
The most recent example of deep learning was when Google used it to create a computer program capable of writing its own code. This was done using a neural network called "Google Brain," which was trained on a massive amount of data from YouTube videos.
This enabled it to learn how programs could be written for itself.
IBM announced in 2015 they had created a computer program that could create music. Also, neural networks can be used to create music. These are known as NNFM, or "neural music networks".
What is the role of AI?
To understand how AI works, you need to know some basic computing principles.
Computers keep information in memory. Computers work with code programs to process the information. The code tells a computer what to do next.
An algorithm is a set or instructions that tells the computer how to accomplish a task. These algorithms are often written using code.
An algorithm could be described as a recipe. A recipe could contain ingredients and steps. Each step may be a different instruction. An example: One instruction could say "add water" and another "heat it until boiling."
What are some examples AI-related applications?
AI can be used in many areas including finance, healthcare and manufacturing. These are just a few of the many examples.
-
Finance - AI is already helping banks to detect fraud. AI can scan millions upon millions of transactions per day to flag suspicious activity.
-
Healthcare – AI helps diagnose and spot cancerous cell, and recommends treatments.
-
Manufacturing - AI in factories is used to increase efficiency, and decrease costs.
-
Transportation – Self-driving cars were successfully tested in California. They are being tested across the globe.
-
Utility companies use AI to monitor energy usage patterns.
-
Education - AI can be used to teach. Students can use their smartphones to interact with robots.
-
Government - AI is being used within governments to help track terrorists, criminals, and missing people.
-
Law Enforcement - AI is being used as part of police investigations. Search databases that contain thousands of hours worth of CCTV footage can be searched by detectives.
-
Defense - AI is being used both offensively and defensively. Artificial intelligence systems can be used to hack enemy computers. In defense, AI systems can be used to defend military bases from cyberattacks.
How does AI impact the workplace
It will change how we work. We can automate repetitive tasks, which will free up employees to spend their time on more valuable activities.
It will improve customer service and help businesses deliver better products and services.
It will help us predict future trends and potential opportunities.
It will enable companies to gain a competitive disadvantage over their competitors.
Companies that fail to adopt AI will fall behind.
How does AI function?
An artificial neural system is composed of many simple processors, called neurons. Each neuron receives inputs form other neurons and uses mathematical operations to interpret them.
Neurons can be arranged in layers. Each layer has a unique function. The first layer receives raw information like images and sounds. Then it passes these on to the next layer, which processes them further. The final layer then produces an output.
Each neuron has a weighting value associated with it. This value gets multiplied by new input and then added to the sum weighted of all previous values. If the result is more than zero, the neuron fires. It sends a signal down to the next neuron, telling it what to do.
This is repeated until the network ends. The final results will be obtained.
Statistics
- By using BrainBox AI, commercial buildings can reduce total energy costs by 25% and improves occupant comfort by 60%. (analyticsinsight.net)
- The company's AI team trained an image recognition model to 85 percent accuracy using billions of public Instagram photos tagged with hashtags. (builtin.com)
- According to the company's website, more than 800 financial firms use AlphaSense, including some Fortune 500 corporations. (builtin.com)
- While all of it is still what seems like a far way off, the future of this technology presents a Catch-22, able to solve the world's problems and likely to power all the A.I. systems on earth, but also incredibly dangerous in the wrong hands. (forbes.com)
- In 2019, AI adoption among large companies increased by 47% compared to 2018, according to the latest Artificial IntelligenceIndex report. (marsner.com)
External Links
How To
How to get Alexa to talk while charging
Alexa, Amazon’s virtual assistant, is able to answer questions, give information, play music and control smart-home gadgets. It can even hear you as you sleep, all without you having to pick up your smartphone!
Alexa allows you to ask any question. Simply say "Alexa", followed with a question. Alexa will respond instantly with clear, understandable spoken answers. Plus, Alexa will learn over time and become smarter, so you can ask her new questions and get different answers every time.
You can also control other connected devices like lights, thermostats, locks, cameras, and more.
Alexa can adjust the temperature or turn off the lights.
Alexa to Call While Charging
-
Open Alexa App. Tap Settings.
-
Tap Advanced settings.
-
Select Speech Recognition
-
Select Yes, always listen.
-
Select Yes, only the wake word
-
Select Yes, and use the microphone.
-
Select No, do not use a mic.
-
Step 2. Set Up Your Voice Profile.
-
You can choose a name to represent your voice and then add a description.
-
Step 3. Step 3.
Speak "Alexa" and follow up with a command
For example: "Alexa, good morning."
Alexa will reply to your request if you understand it. Example: "Good Morning, John Smith."
Alexa won’t respond if she does not understand your request.
After these modifications are made, you can restart the device if required.
Notice: If you modify the speech recognition languages, you might need to restart the device.